Four Emerging Trends in Data Science and Machine Learning
Updated: Feb 20
Stay Ahead of the Curve: Key Trends in Data Science and ML You Can't Ignore
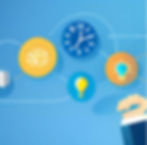
The field of data science and machine learning (ML) is undergoing significant changes with the advancements in AI. These changes are shaping a more flexible and agile landscape, resembling a Swiss Army knife model. In a recent Gartner webinar titled Data Science and Machine Learning Emerging Trends You Can't Ignore, four key trends were identified:
1. ML Platforms Evolve into Digital Ecosystems:
Modular ML platforms, previously operating separately from data management and governance systems, are now transitioning into comprehensive ecosystems. These ecosystems consolidate various components, including data management and governance, data operations, data fabric, and multiple analytics such as AI, ML, stream analytics, and self-analytics. This consolidation enhances flexibility, agility, productivity, and cost-effectiveness in terms of implementation, integration, and maintenance. It is projected that, by 2025, 55% of IT will adopt these data ecosystems, resulting in a 40% reduction in the vendor landscape.
2. Blurring Boundaries between Data Scientists and Developers:
The traditional segregation between software engineers and data scientists is diminishing. As data and ML become integral to products and services, every application or product becomes data-informed and analytics-driven. Developers must understand data and insights, while data scientists need to contribute to the development of end products. The merging of these two functional groups is essential. ML-powered code editors, where code and data intertwine, enable both data scientists and developers to optimize code generation using a combination of natural language and coding practices. It is estimated that, by 2027, 50% of developers will utilize ML-powered coding tools, a significant increase from the current less than 5% adoption rate.
3. Focus on Building Responsible AI:
The focus of ML has shifted beyond merely building models and driving business operations. It now encompasses the responsible design, development, and deployment of AI systems. Ensuring good intentions, empowering businesses, and considering the fair impact on customers and society have become integral parts of ML functions. This integration promotes trust and scalability of AI. Responsible AI is now an essential aspect of data science and ML platforms, as well as cloud ecosystems. The outcome must be fair, transparent, explainable, accountable, socially beneficial, and secure. It is predicted that, by 2025, the concentration of pretrained AI models among 1% of AI vendors will elevate responsible AI as a societal concern.
4. Transition from Predictive Models to Generative AI:
ML is no longer limited to understanding patterns, providing diagnostics , and making predictions. It has now entered the decision-making process, leading to the era of decision intelligence. ML is increasingly automating decision-making tasks and generating various types of content, such as reports, articles, answers to questions, and even code writing.
These four emerging trends in data science and ML indicate the direction in which the field is progressing, promising a future where data-driven insights, responsible AI, and automated decision-making play vital roles.
As every company becomes a technology company and every business embraces AI, ignoring these trends will put your company at a disadvantage in the competitive landscape.

May you grow to your fullest in AI & Data Science!
*** Please DOWLOAD the FREE document, Find your signature vision questionnaires so you use it to help you find your life vision and mission.
>> Subscribe Grow to Your Fullest and get your Free Download
>> Contact Grow to Your Fullest
>> Read on Substack
>> Watch on YouTube
>> Connected on Facebook